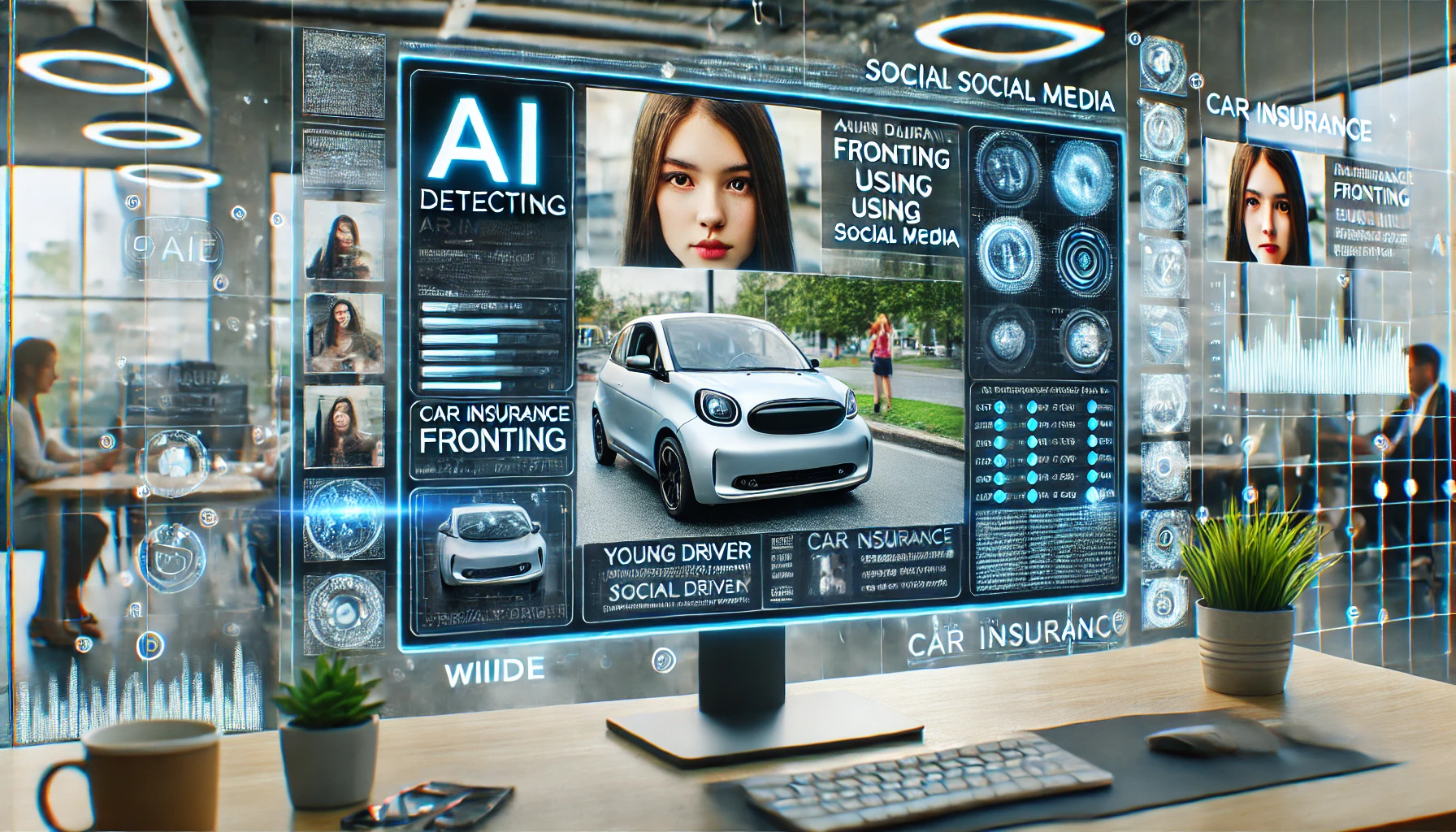
What is Fronting?
Fronting is a type of car insurance fraud where the main driver of the vehicle, typically a younger or less experienced driver, is listed as an additional or named driver on the policy, while a more experienced driver, such as a parent, is falsely declared as the primary driver.
This deceitful practice is used to obtain lower insurance premiums, as insurance companies often charge higher rates for high-risk drivers. However, fronting is illegal and can lead to severe consequences, including voided insurance coverage, fines, and legal action if discovered.
Using AI to Detect Fronting
AI, or Artificial Intelligence, is the simulation of human intelligence processes by computer systems. AI can automate complex tasks, improve efficiency, and provide insights through data analysis, leading to enhanced decision-making and productivity.
🤖 AI Social Media Fronting Detection
- Profile Analysis: AI analyzes social media profiles for driver inconsistencies.
- Behavioral Patterns: AI tracks driving-related posts and patterns.
- Location Data: AI uses geotags to match car movements with drivers.
- Social Connections: AI examines social interactions and tags involving the car.
- Cross-Referencing Data: AI cross-references social media with other data sources for driver ID.
- Sentiment Analysis: AI gauges attitudes and behaviors from posts and comments about cars & insurance.
Social media platforms are treasure troves of personal information that can provide insights into the real lives and behaviors of individuals. Car insurance companies are increasingly utilizing AI to tap into this vast resource to detect fronting fraud. Here are some ways AI and social media are combined to uncover such fraudulent activities:
Driver profile analysis:
AI algorithms can analyze social media profiles to gather information about the daily activities, lifestyle, and behavior of both the primary and named drivers on a policy. By examining posts, photos, and check-ins, AI can identify inconsistencies between the declared primary driver and the actual person who seems to be using the vehicle most frequently.
Driver behavioral patterns:
AI can track and analyze the frequency and timing of posts related to driving. For example, if the named driver frequently posts about commuting to work or school, while the primary driver has minimal driving-related content, this can raise a red flag. Patterns such as posts about long trips, weekend getaways, or late-night drives by the named driver can indicate they are the true primary driver.
Car location data:
Many social media posts include geolocation tags. AI can analyze this location data to see who is most often in the vicinity of the car's usual locations, such as home, work, or school. If the named driver’s geolocation data frequently matches the car’s movements, it suggests they might be the primary user of the vehicle.
Driver social connections:
AI can also look at social connections and interactions. For example, if the named driver is tagged in multiple posts or photos involving the car, this can be a strong indicator of fronting. Comments and interactions related to driving experiences or the vehicle can also provide clues about the actual primary driver.
Cross-referencing driver data:
AI systems can cross-reference social media data with other information sources such as telematics, public records, and insurance claims to verify the authenticity of the primary driver’s identity. Discrepancies between what is reported in the insurance application and the evidence gathered from social media can prompt further investigation.
Driver sentiment analysis:
AI can perform sentiment analysis on posts and comments to gauge the attitudes and behaviors of the drivers. Posts expressing excitement about driving a new car or frequent mentions of driving-related activities by the named driver can suggest fronting. Negative sentiments or complaints about high insurance costs by the primary driver can also be indicators of potential fronting fraud.
Conclusion
By integrating AI with social media analysis, car insurance companies can more effectively detect and prevent fronting. This approach not only helps in identifying fraudulent claims but also ensures that insurance premiums are accurately assessed based on the true risk profile of the policyholders. The use of social media data, combined with AI's analytical capabilities, provides a powerful tool for maintaining the integrity of the insurance industry.
FAQ: AI fronting detection
Yes, AI can analyze data in real-time, using telematics and other real-time data sources to immediately identify suspicious driving behavior patterns that may indicate fronting.
AI differentiates between fronting and legitimate shared driving by analyzing patterns over time. If the named driver consistently uses the vehicle in ways typical of a primary driver, it may indicate fronting. Shared driving usually shows more balanced usage between drivers.
Yes, AI looks for red flags such as the named driver having a higher driving frequency, social media posts indicating regular vehicle use, discrepancies in driving patterns, and unusual claims history.
AI can be applied to detect fronting for most types of personal vehicles, including cars, trucks, and motorcycles. However, the effectiveness may vary depending on the availability and quality of data.
Insurance companies implement AI systems by integrating various data sources, training machine learning models on historical data, and continuously updating algorithms to adapt to new fraud tactics.
The use of AI to detect fronting does not directly increase insurance premiums. Instead, it helps ensure that premiums are accurately based on risk, potentially lowering costs for honest policyholders.
Yes, AI systems are designed to handle large volumes of data efficiently, using advanced algorithms and computing power to process and analyze information quickly.
AI improves the speed of detecting fronting by automating data analysis, quickly identifying patterns and anomalies that would take human investigators much longer to uncover.
While AI is highly effective, it is not infallible. It may sometimes flag legitimate cases as suspicious or miss sophisticated fraud. Human oversight is still necessary to review and confirm AI findings.
If you are wrongly flagged for fronting, you should contact your insurance provider, provide any requested documentation, and cooperate with their investigation to clear up the misunderstanding.